VideoMind: Revolutionizing Temporal-Grounded Video Reasoning with Chain-of-LoRA
Explore VideoMind's innovations in temporal-grounded video reasoning with agentic workflows and Chain-of-LoRA strategy for multimodal AI.
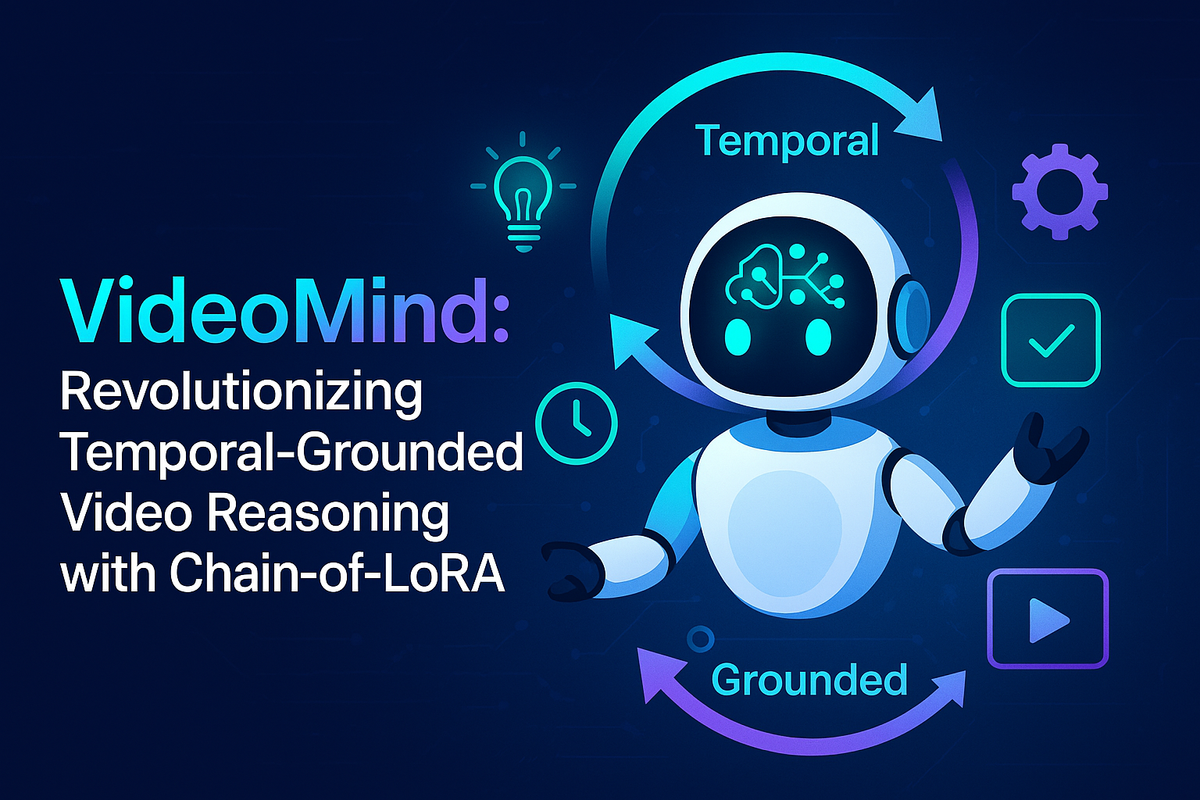
Ever wondered how AI could tackle the complexities of video reasoning, like understanding long-form videos or pinpointing key moments in a sequence? That’s where VideoMind comes in. This groundbreaking model redefines video understanding by leveraging innovative strategies like agentic workflows and the Chain-of-LoRA technique. Let’s break it down.
Challenges in Video Understanding
Working with videos isn’t like handling static images. Videos are dynamic, with events unfolding over time. To make sense of this, AI needs to grasp temporal relationships—basically, the “when” and “how” moments connect. Current methods excel at answering simple questions about images or short clips but struggle with tasks requiring deeper reasoning and precise localization within longer videos.
- Multi-modal reasoning: Combining text, visuals, and their contextual interplay.
- Temporal grounding: Pinpointing specific moments within a timeline.
- Interpretability: Explaining how decisions are made, especially with long-form videos.
These gaps highlight the need for smarter systems that do more than process frames. Enter VideoMind.
Introduction to VideoMind
VideoMind steps up to tackle these issues head-on. Developed by researchers from Hong Kong Polytechnic University and the National University of Singapore, VideoMind introduces two game-changing concepts:
- Agentic Workflow: This breaks down video reasoning into specialized roles:
- Planner: The brain of the operation, deciding what needs to happen next.
- Grounder: Pinpoints key timestamps based on the query.
- Verifier: Checks the validity of identified intervals with a simple “Yes” or “No.”
- Answerer: Generates answers using either cropped video segments or the complete video.
- Chain-of-LoRA Strategy: A smart technique for role-switching using lightweight LoRA adaptors, which keeps the process efficient without requiring multiple bulky models.
Take a look at Figure 1 below—it illustrates how VideoMind’s architecture brings these pieces together.
The diagram above shows how the user interacts with VideoMind components to perform tasks like query processing, timestamp localization, and answer generation.
Performance Benchmarks
So, how does VideoMind stack up against other models? Spoiler: it’s pretty impressive.
Key Highlights:
- Lightweight Efficiency: The 2B version of VideoMind outperforms much larger models like InternVL2-78B and Claude-3.5-Sonnet in most metrics. Even GPT-4o struggles to keep up with VideoMind's 7B version.
- Zero-Shot Capabilities: Without additional training, VideoMind delivers top-tier results on benchmarks like NExT-GQA, outperforming fine-tuned solutions.
- General Video QA: Models excel in tasks requiring cue segment localization across datasets like Video-MME (Long), MLVU, and LVBench.
The diagram above maps out the interconnections between VideoMind’s components, showcasing the flow from query input to answer generation and validation.
Conclusion and Future Directions
VideoMind isn’t just solving today’s problems; it’s paving the way for tomorrow’s advancements in multimodal AI. By combining agentic workflows with the Chain-of-LoRA strategy, it offers a glimpse into what’s next for complex video understanding.
What’s the takeaway? VideoMind is setting new standards for interpreting long-form videos, offering precise, evidence-based answers with unmatched efficiency. But this is just the start—the future holds even more exciting possibilities for multimodal agents.
If you want to dig deeper, check out the Paper and Project Page.
FAQ
Q: What is VideoMind and how does it improve video reasoning?
A: VideoMind is an AI model designed for temporal-grounded video understanding. It uses an agentic workflow and Chain-of-LoRA strategy to analyze long-form videos efficiently.
Q: How does the Chain-of-LoRA strategy work in VideoMind?
A: Chain-of-LoRA dynamically activates role-specific adaptors during inference, enabling seamless role-switching without heavy computational overhead.
Q: What are the components of VideoMind’s agentic workflow?
A: The workflow includes the Planner, Grounder, Verifier, and Answerer—each specialized for tasks like timestamp localization and answer generation.
Q: How does VideoMind perform compared to other models?
A: VideoMind outperforms many larger models in benchmarks, showing exceptional zero-shot and general video QA capabilities.
Q: What challenges does VideoMind address in video understanding?
A: It tackles issues like temporal dynamics, interpretability, and reasoning over long-form videos to deliver evidence-based answers.